Causal inference is gaining significant attention in the world of data science and predictive analytics, especially in the context of business decision-making. While machine learning has revolutionized the way businesses forecast trends and make predictions, causal inference provides an entirely different approach by focusing on understanding WHY something happens, rather than merely predicting outcomes based on historical data.
In this article, we’ll explore what causal inference is, how it differs from traditional machine learning methods, and its key benefits for businesses. We will also dive into common applications of causal inference in business and how no-code tools can simplify this advanced analysis for broader use.
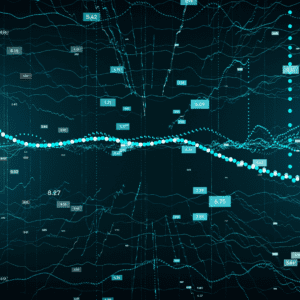
What Is Causal Inference?
Causal inference is the process of determining whether a cause-and-effect relationship exists between variables. Unlike traditional machine learning, which is often focused on correlations and predictive power, causal inference seeks to establish causality. For example, instead of just predicting that an increase in marketing spend will likely lead to an increase in sales, causal inference would answer the question: Did the marketing spend actually cause the increase in sales?
Machine Learning vs Causal Inference Difference
Machine Learning
- Finds patterns in data, but does not understand why those patterns exist.
- Analyzes historical data to predict future trends, but these predictions are often based on correlations and don’t explain the underlying mechanisms
- Requires large datasets and uses past patterns to make future predictions
Causal Inference
- Goes beyond ML patterns to establish whether changes in one variable actually cause changes in another
- Answers the critical “why” question, about the mechanisms behind the future trends
- Works with smaller datasets if the focus is on experimental or quasi-experimental designs, the quality and design of the data collection process are often more critical than the volume of data
Why Is Causal Inference Important in Business?
For businesses looking to optimize strategies and allocate resources effectively, causal inference provides several unique benefits that go beyond traditional machine learning methods:
– Actionable Insights: Unlike machine learning models, which predict “what will happen,” causal inference answers “what will happen if I take this action?” This helps businesses understand the direct impact of decisions and interventions.
– More Accurate Decision-Making: By establishing cause-and-effect relationships, businesses can better predict the outcomes of specific actions, making decisions based on solid evidence rather than correlations that might not be meaningful.
– Optimization of Resources: With causal insights, businesses can allocate resources more efficiently. For example, knowing which marketing channel causes more sales can help companies direct their budgets more effectively.
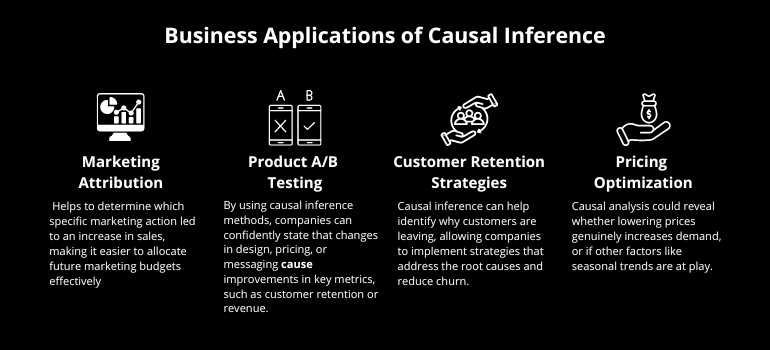
How No-Code Tools Empower Causal Inference
The rise of no-code platforms has democratized data science and analytics, allowing non-technical users to perform complex tasks like machine learning without needing to write code. This trend is extending to causal inference, with several no-code platforms now offering built-in tools for causal analysis.
No-code tools like NextBrain AI allow business analysts and decision-makers to design experiments, run A/B tests, and perform causal analysis without the need for a deep understanding of statistics or programming. This accessibility allows businesses of all sizes to harness the power of causal inference to drive better decision-making.
Conclusion: Why Businesses Need Both Machine Learning and Causal Inference
While machine learning remains an indispensable tool for prediction and forecasting, causal inference fills an important gap by providing actionable insights that go beyond correlation. By understanding why something happens, businesses can make more informed decisions and optimize their resources more effectively. No-code tools make this technology accessible to a wider audience, enabling businesses to integrate causal inference into their decision-making processes with ease.
Incorporating both machine learning and causal inference into your analytics strategy offers the best of both worlds: predictive power to forecast future outcomes and causal insights to understand the drivers behind those outcomes. By using these complementary approaches, businesses can gain a deeper understanding of their data and make smarter, evidence-based decisions.
To explore the potential insights AI can extract from your data, schedule a demo of NextBrain AI today, and let us show its capabilities with your data firsthand.