Use of AI is now spread over many fields, and physics is no exception.
Thus, scientists from MIT and the University of Basel have recently employed generative artificial intelligence to tackle difficult questions in physics, particularly in classifying phases of physical systems.
This advanced method could significantly enhance the investigation of novel materials. Let’s see in detail how it works.
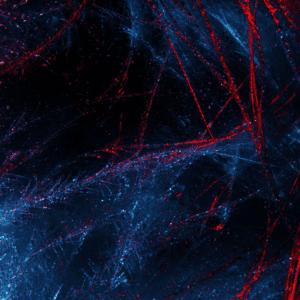
The Research of Phase Transitions
The researchers applied AI to study phase transitions in newly developed materials. Phase transitions, such as when water freezes into ice, result in significant changes in properties like density and volume. While these transitions in common substances are well understood, those in novel materials or complex systems remain a critical area of research. Recognizing and quantifying these transitions in unknown systems is often a challenge due to limited data.
The researchers from MIT and the University of Basel have developed a machine-learning framework that employs generative AI to automatically map phase diagrams for new physical systems. This physics-informed approach is more efficient than traditional, manual techniques, which rely heavily on theoretical expertise and large, labeled datasets.
Traditional methods for detecting phase transitions involve identifying an “order parameter” that changes during the transition. For instance, water transitioning to ice involves the temperature dropping below 0 degrees Celsius, with the order parameter being the proportion of water molecules in a crystalline state. AI models, like generative classifiers, can now handle this task more efficiently and without human bias.
Application in Advanced Materials and Quantum Systems
The new AI framework could significantly impact the study of thermodynamic properties in novel materials and the detection of quantum entanglement. This technique opens the door to discovering previously unknown phases of matter autonomously, streamlining the scientific discovery process.
Advantages of Generative Models
Generative models, which estimate probability distributions to generate new data points, offer significant advantages in constructing classifiers. The MIT team’s approach involves using these models to incorporate known physical system properties deeply into the machine-learning framework, enhancing the accuracy and efficiency of phase detection.
The potential applications of this generative AI approach extend beyond phase transitions. It could be used to solve various binary classification tasks in physical systems, detect quantum entanglement, and even improve large language models by optimizing parameter tuning. Future research aims to establish theoretical guarantees on the number of measurements needed to detect phase transitions effectively.
As AI becomes increasingly integrated into various aspects of our lives, avoiding it puts you at a disadvantage. Our AI-based data analytics platform, NextBrain AI, is designed to help every business use the benefits of AI. Schedule your demo today to discover the insights AI can reveal from your data.