” … as far as the propositions of mathematics refer to reality, they are not certain; and as far as they are certain, they do not refer to reality.”
Albert Einstein
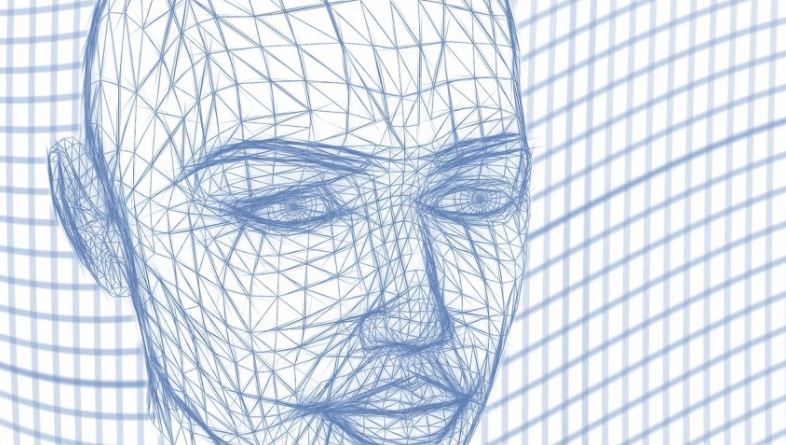
Essentially, synthetic data technology empowers practitioners to effortlessly fabricate the data they seek, instantly and abundantly, customized to their exact specifications. For example, a manufacturing company is implementing a new process that requires a lot of experimentation. Running such tests demands significant resources in terms of time and money, particularly when our aim is to ensure the seamless functionality of these implementations. The use of synthetic data can help reduce this effort, speeding up the go-to-market strategy. This way, it looks like the use of synthetic data is the silver bullet for any business.
But there are some critics who, in the absence of enough successful business cases, claim that this technology has some issues. They claim that one of the most important insights of data analysis is estimating the uncertainty associated with our conclusions, which can be influenced by the amount of data available. Thus, introducing synthetic data might artificially enhance our confidence in our analysis, leading us to make wrong decisions.
Uncertainty has been the cornerstone of decision sciences for years, to the point that it has cornered such basic concepts as the study of the nature of the data or the understanding of its underlying global structure. For this reason, modern mathematicians and data scientists are moving to a new approach based on a basic idea: data has shape, and shape has meaning. And this meaning is at least as valuable as the uncertainty associated with its use.
As a conclusion, embracing novel perspectives on data is acute in our quest to adopt new opportunities like synthetic data in our decision-making processes.