Understanding Marketing Mix Modelling in Today’s Digital Age
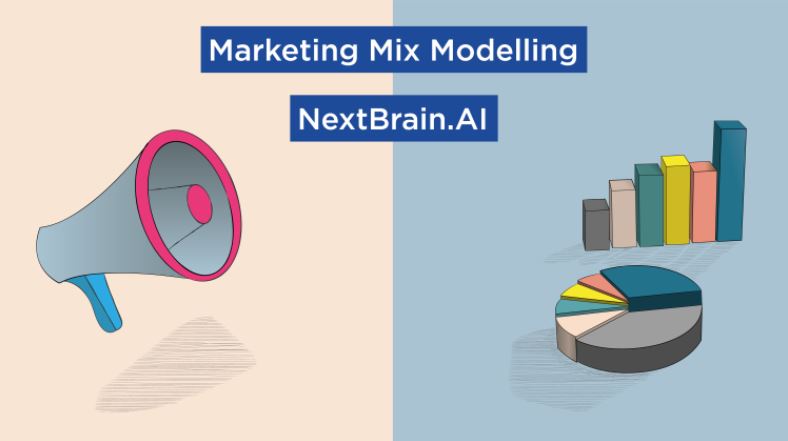
Marketing Mix Modelling (MMM) is currently a hot topic for numerous reasons. In the digital era, it faces critical challenges that brands must navigate. The proliferation of platforms has complicated the task of establishing a brand’s voice and personality. Simultaneously, the exponential growth of social networks has empowered consumers, leading to the emergence of countless new niches and audiences. As a result, building a meaningful relationship between brands and their audiences, along with accurately measuring its impact, has become increasingly formidable. The days of enduring relationships between brands and consumers have given way to rapidly shifting scenarios, necessitating a refresh in Marketing Mix Modelling strategies.
Key Challenges in Marketing Mix Modelling
この複雑な状況は、以下を含むいくつかの課題を提示します:
Data Quality
With shorter campaigns targeting smaller segments, the availability of data has decreased. Traditional statistical models and standard machine learning algorithms rely on large datasets. To address this limitation, new マーケティングミックスモデリング techniques such as Bayesian models, Graph models, and causally explainable Deep Learning models need to be adopted. Synthetic data, which offers equivalent output sets to the input data, can also help generate larger sample datasets suitable for machine learning algorithms.
Funnel Effects
In a multi-channel environment where brand personality is built across numerous channels, funnel effects play a significant role. As the number of channels increases, spanning online and offline platforms, Marketing Mix Modelling becomes more intricate, and the interplay between different channels becomes inevitable. Existing MMM models often struggle to comprehend this mix, leading to the undervaluation of certain channel effects. Understanding and accounting for funnel effects become crucial when establishing a brand personality across multiple channels.
Selection Bias and Causality
The relationship between advertising and demand is no longer straightforward due to the abundance of channels, media, and audiences. Selection bias arises when an input media variable correlates with one or more hidden demand factors. Failure to identify these factors may result in erroneous interpretations of media contributions. New MMM models need the capability to incorporate other variables to accurately assess the effectiveness of different campaigns.
Measuring Creativity
デジタル領域において、消費者は非常に関与の高いリスナーとなっています。ブランドが顧客とコミュニケーションを取れない場合、別のブランドがその機会を奪います。ブランドは常に新しいクリエイティブ戦略を開発し、その影響を測定する効率的な方法を見つける必要があります。MMMモデルは、チャネルの貢献を測定するだけでなく、異なるクリエイティブアプローチの効果も評価する必要があります。
これらの課題に対処し、革新的な技術を取り入れることで、進化するマーケティング環境の要求に応えるためにマーケティングミックスモデリングの未来を形成することができます。
The Future of Marketing Mix Modelling
To stay competitive in this dynamic environment, brands must leverage advanced tools and methodologies. NextBrain AI offers a no-code machine learning platform that can help businesses revolutionize their Marketing Mix Modelling strategies. By utilizing our solutions, brands can gain deeper insights into their marketing performance and make informed decisions.
Explore more about our solutions to enhance your marketing effectiveness. Stay ahead of the competition and unlock the potential of your marketing strategy.
For more insights on marketing and technology, read more on our blog and discover the latest trends and strategies.